LLama factory lora微调qwen部署 brings a revolutionary approach to fine-tuning and deploying AI models, making it an essential tool for tech enthusiasts and developers. This guide will walk you through the exciting advancements in LoRA tuning and Qwen deployment, ensuring that you harness the full potential of LLaMA Factory’s capabilities.
Whether you’re looking to enhance model performance or streamline deployment processes, understanding the intricacies of “lora微调qwen部署” can significantly boost your AI projects. In this blog post, we’ll delve into the latest techniques and practical tips to optimize your use of LLaMA Factory.
Understanding LoRA Tuning: A Comprehensive Guide
LoRA tuning, or Low-Rank Adaptation, is a technique used to enhance the efficiency of AI models. It focuses on adjusting specific model parameters to improve performance while keeping computational requirements low. This method is especially useful for fine-tuning large language models like Qwen, as it allows for significant improvements without the need for extensive computational resources. LoRA tuning is a key feature of LLaMA Factory, offering users a way to optimize their models effectively.
In “lora微调qwen部署,” LoRA tuning plays a crucial role in maximizing model performance. By carefully adjusting parameters and leveraging LoRA’s capabilities, users can achieve better results and more efficient operations. Understanding how LoRA tuning works and applying it correctly can lead to substantial gains in model accuracy and responsiveness. This comprehensive guide will help you grasp the fundamentals of LoRA tuning and how to apply it to your projects using LLaMA Factory.
Why “lora微调qwen部署” Matters for Modern AI
“Lora微调qwen部署” is essential for the advancement of modern AI applications. This process involves fine-tuning the Qwen model using LoRA techniques and deploying it effectively. The significance of this process lies in its ability to adapt models to specific tasks, improving their performance and accuracy in real-world scenarios. Fine-tuning with LoRA allows for more precise control over model behavior, which is crucial for developing sophisticated AI solutions.
The benefits of “lora微调qwen部署” extend beyond mere performance improvements. It also contributes to the overall efficiency of AI systems, reducing computational costs and enhancing operational effectiveness. For developers and researchers, mastering this technique means being able to create more capable and adaptable AI models. This is why understanding and implementing “lora微调qwen部署” is vital for anyone involved in the field of AI.
How to Start with LLaMA Factory for LoRA Fine-Tuning
Getting started with LLaMA Factory for LoRA fine-tuning is a straightforward process. Begin by downloading the latest version of the LLaMA Factory framework from GitHub. Once downloaded, set up your environment by creating a virtual environment and installing the necessary dependencies. This step is crucial for ensuring that all required packages are available and properly configured.
Next, follow the setup instructions provided in the LLaMA Factory documentation. This will guide you through configuring your settings for LoRA fine-tuning. Make sure to review the configuration options and adjust them according to your specific needs. With LLaMA Factory, you can start fine-tuning your model with ease, leveraging its advanced features to achieve optimal results. The framework’s user-friendly interface and detailed guides make the initial setup and fine-tuning process efficient and accessible.
Step-by-Step Guide to Deploying Qwen Models with LLaMA Factory
Deploying Qwen models using LLaMA Factory involves a series of well-defined steps. Begin by preparing your Qwen model for deployment. This includes ensuring that the model is correctly fine-tuned and ready for integration. Next, use the deployment tools provided by LLaMA Factory to incorporate the model into your application. This step involves configuring various parameters and settings to ensure smooth operation.
Once the model is integrated, conduct thorough testing to verify its performance and functionality. This includes checking for any issues or discrepancies and making necessary adjustments. LLaMA Factory provides comprehensive documentation and support to assist you throughout the deployment process. By following these steps, you can effectively deploy your Qwen model and leverage its capabilities for your AI projects.
Maximizing Efficiency with LoRA in LLaMA Factory
To maximize efficiency with LoRA in LLaMA Factory, it is essential to focus on several key strategies. Start by optimizing the configuration settings for LoRA fine-tuning. Adjust parameters based on your specific needs and the requirements of your model. Regularly review and refine these settings to ensure optimal performance.
Incorporate best practices for resource management to reduce computational costs. LoRA’s ability to enhance model performance while maintaining efficiency is a significant advantage. By effectively managing resources and optimizing configurations, you can achieve better results and more efficient operations. LLaMA Factory’s features and tools will help you implement these strategies effectively, leading to improved efficiency in your AI projects.
Common Challenges in “lora微调qwen部署” and How to Overcome Them
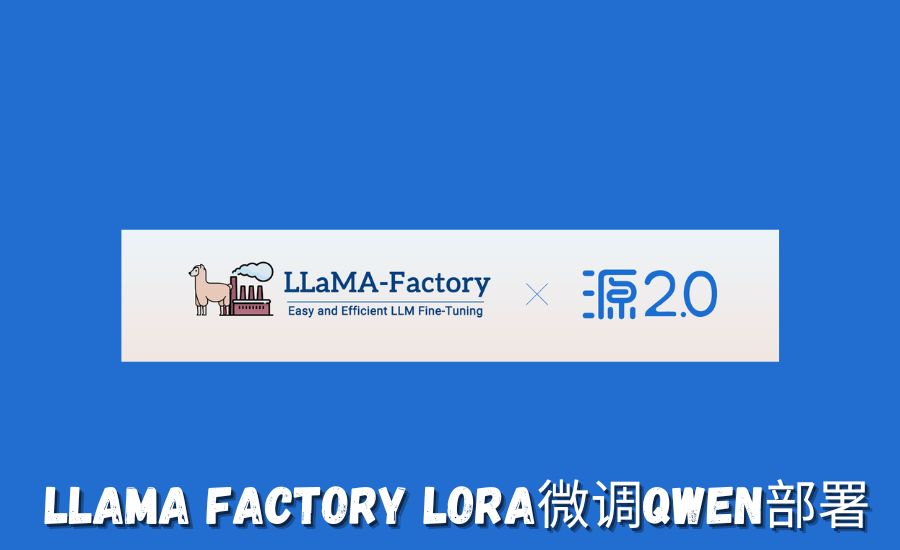
Deploying “lora微调qwen部署” can present several common challenges. Issues may include configuration errors, performance discrepancies, and compatibility problems. To overcome these challenges, start by carefully reviewing your setup and configuration. Ensure that all parameters and settings are correctly applied.
Utilize available troubleshooting resources, such as documentation and community forums, to address any issues. Regularly test your deployment and make necessary adjustments based on feedback and performance results. By addressing these challenges proactively, you can ensure a successful deployment and optimal performance for your Qwen model.
Comparing LoRA Tuning Techniques: What You Need to Know
When comparing LoRA tuning techniques, it is important to evaluate various factors, including efficiency, accuracy, and computational requirements. Different techniques may offer distinct advantages, so it is crucial to choose the one that best fits your specific needs and goals.
Consider the performance of each technique in real-world scenarios to determine its effectiveness. By understanding the strengths and limitations of different LoRA tuning methods, you can make informed decisions about which technique to use for your AI projects. This comparison will help you select the most suitable approach for achieving the best results.
Best Practices for Fine-Tuning Models with LLaMA Factory
Following best practices for fine-tuning models with LLaMA Factory is essential for achieving optimal results. Start by using high-quality data for training and validation. This will ensure that your model is well-prepared for fine-tuning and capable of delivering accurate performance.
Adjust parameters and settings based on your specific requirements and regularly monitor performance. Incorporate feedback and improvements into your fine-tuning process to maintain high standards. By adhering to these best practices, you can enhance your model’s performance and achieve successful outcomes in your AI projects.
You May Also Like: Naka-technologies-events
Advanced Strategies for Qwen Deployment
Implementing advanced strategies for Qwen deployment can significantly enhance model performance. Explore methods such as custom configurations and optimizations to achieve better results. These strategies involve adjusting various settings and parameters to align with your project goals.
Carefully implement these advanced techniques to ensure compatibility and effectiveness. By leveraging sophisticated strategies, you can achieve improved performance and efficiency in your Qwen model deployment. Advanced approaches can lead to substantial gains in model capabilities and overall success.
Troubleshooting “lora微调qwen部署” Issues in LLaMA Factory
Troubleshooting issues related to “lora微调qwen部署” in LLaMA Factory is crucial for maintaining smooth operation. Common problems may include setup errors, performance issues, and compatibility challenges. Begin by reviewing your configuration and setup to identify any potential issues.
- Check Configuration: Ensure all parameters and settings are correctly applied.
- Utilize Resources: Use troubleshooting guides and community support.
- Regular Testing: Conduct thorough tests to identify and resolve issues.
Utilize available troubleshooting resources, such as documentation and community forums, to address any issues. Stay updated on the latest fixes and solutions to address any challenges effectively. By troubleshooting issues promptly, you can ensure optimal performance and successful deployment of your Qwen model.
Case Studies: Successful Implementations of LLaMA Factory
Case studies of successful implementations of LLaMA Factory provide valuable insights into effective usage and strategies. These examples showcase how different projects have leveraged LLaMA Factory to achieve their goals. Reviewing these cases can offer practical guidance and inspiration for your own projects.
Analyze the approaches and techniques used in these successful implementations to enhance your understanding of best practices. Learning from real-world examples will help you apply similar strategies to your own AI projects, leading to better outcomes and greater success.
The Future of AI Fine-Tuning with LLaMA Factory
The future of AI fine-tuning with LLaMA Factory looks promising as technology continues to advance. New features and improvements will further enhance model performance and capabilities. Staying informed about the latest developments will help you keep your projects at the cutting edge of AI technology.
Embrace emerging technologies and techniques to stay ahead in the field. The ongoing evolution of LLaMA Factory will shape the future of AI fine-tuning, offering new opportunities and advancements for your projects.
Integrating LLaMA Factory with Other AI Tools
Integrating LLaMA Factory with other AI tools can enhance the functionality and performance of your projects. Explore ways to combine LLaMA Factory with tools like TensorFlow or PyTorch for more versatile AI solutions. Integration can lead to more robust and comprehensive AI systems.
Follow best practices for integration to ensure compatibility and optimal performance. By combining tools effectively, you can achieve better results and more powerful AI solutions. Integration strategies will help you leverage the strengths of different tools and enhance your AI projects.
Optimizing Performance: Tips for LoRA Tuning and Qwen Deployment
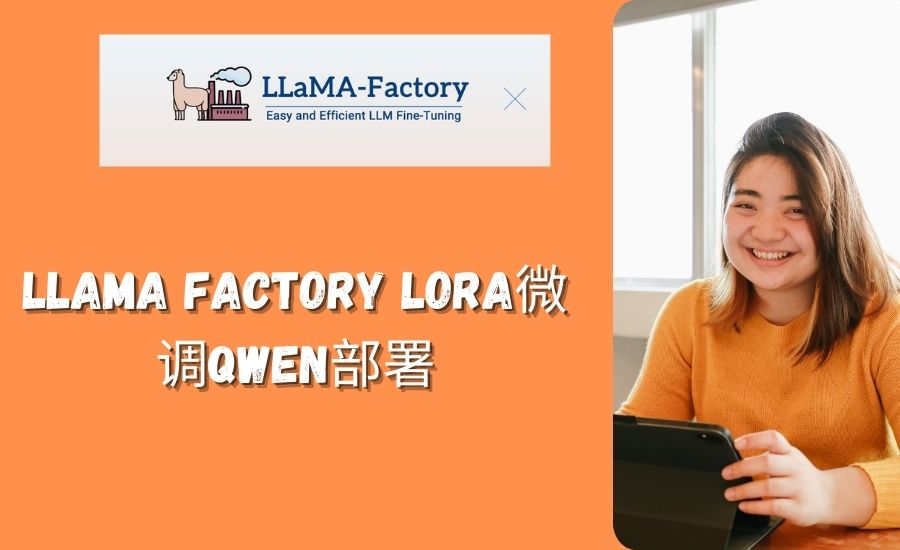
To optimize performance for LoRA tuning and Qwen deployment, focus on key strategies like parameter adjustments and resource management. Regularly review and refine your settings to achieve the best outcomes for your AI models.
Utilize available tools and techniques to enhance efficiency and accuracy. Effective optimization will lead to improved performance and more successful deployments. By applying these tips, you can achieve better results and maximize the potential of your LoRA tuning and Qwen deployment.
User Reviews and Feedback on LLaMA Factory
User reviews and feedback provide important insights into LLaMA Factory’s effectiveness and usability. Reading reviews can help you understand the strengths and areas for improvement of the framework. Feedback from other users can guide your implementation strategies and usage.
Consider both positive and negative feedback to get a balanced view. User reviews offer valuable information that can inform your decisions and help you make the most of LLaMA Factory. By taking into account user experiences, you can enhance your own use of the framework.
Conclusion
In conclusion, LLaMA Factory is a powerful tool that makes working with AI models easier and more efficient. By using LLaMA Factory, you can fine-tune and deploy models like Qwen with less hassle. Its features help you get better results faster, which is great for both beginners and experts in AI. If you follow the tips and best practices mentioned, you’ll see how much LLaMA Factory can improve your projects.
As AI technology keeps advancing, LLaMA Factory will keep getting better, offering new ways to enhance your models. By staying updated and using the latest features, you can keep your AI projects on the cutting edge. So, if you want to get the most out of your AI models, give LLaMA Factory a try and see how it can make your work easier and more effective!
FAQ,s
Q: What is LLaMA Factory?
A: LLaMA Factory is a framework designed to simplify the process of fine-tuning and deploying large language models like LLaMA, BLOOM, and Qwen. It helps users easily adjust and improve AI models for specific tasks.
Q: How does LoRA tuning work with LLaMA Factory?
A: LoRA tuning is a technique that adjusts certain layers of a model to improve its performance. In LLaMA Factory, this method is used to fine-tune models efficiently, allowing for better results with less computational effort.
Q: What is the purpose of “lora微调qwen部署”?
A: The term “lora微调qwen部署” refers to using LoRA tuning to fine-tune and deploy the Qwen model. This process helps in optimizing Qwen for specific applications or tasks by making precise adjustments.
Q: How can I start using LLaMA Factory for LoRA fine-tuning?
A: To start with LLaMA Factory, download the framework from its GitHub page, create a suitable environment, and follow the setup instructions. You can then begin fine-tuning your models using the provided tools and scripts.
Q: What are common issues with “lora微调qwen部署”?
A: Common issues include errors related to tensor probabilities or configuration problems. Checking your setup, updating the framework, and ensuring compatibility with your hardware can help resolve these issues.
Q: How can I optimize performance with LoRA in LLaMA Factory?
A: To optimize performance, ensure you are using the latest version of LLaMA Factory, follow best practices for tuning, and adjust parameters based on your specific needs. Regularly review documentation and community tips for improvements.
Q: Where can I find support or more information about LLaMA Factory?
A: For support, check the LLaMA Factory GitHub page for documentation and issues. You can also find discussions and additional tips from the user community in forums and related online groups.
Stay Connected with DenverInsiders for the Latest Blogs
Leave a Reply